Digitization in battery research
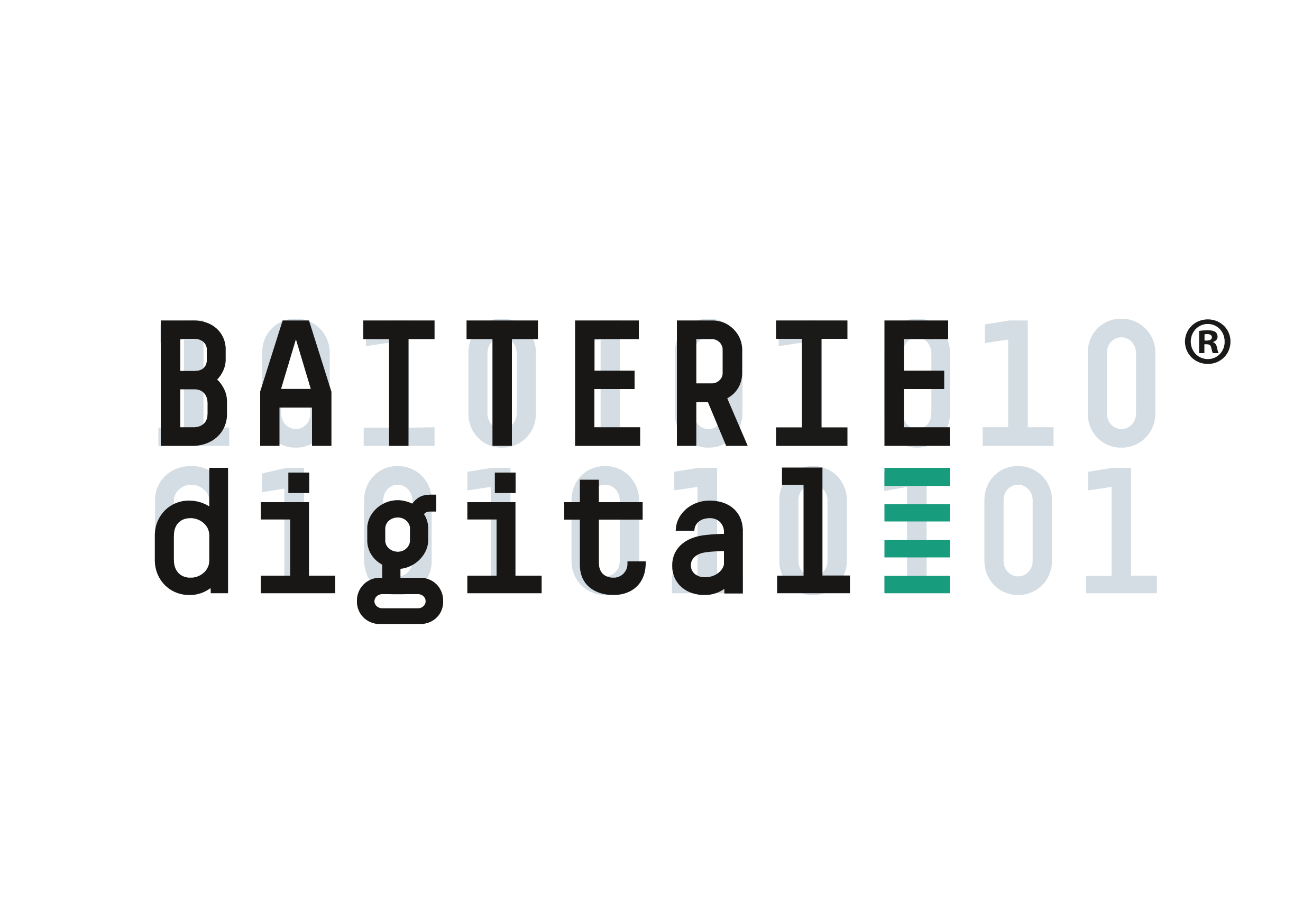
The digitization of empirical research data and the development of digital workflows for automated data processing using models and intelligent algorithms have increasingly become the focus of battery research in recent years. The development and technical implementation of a comprehensive digitization strategy for the efficient and sustainable use of battery data requires the complete digitization of all methods and processes for data generation and processing, as well as systematic management of the data throughout its entire life cycle. The digitization strategy of battery data is addressed at Fraunhofer IFAM in BATTERIEdigital®.
Fraunhofer IFAM aims to use the registered trademark BATTERIEdigital® to drive the digital transformation of battery research by taking a holistic view of all topics from experimental data generation, intelligent data processing and analysis, to the development of automated workflows. By bringing together comprehensive competencies and innovative technologies from the fields of materials science, electrochemical energy storage, data analytics and informatics, digital tools and services for cell diagnostics as well as for the identification and characterization of novel materials for battery cells are developed in BATTERIEdigital®.
A particular application focus of BATTERIEdigital® lies in the intelligent determination and prediction of the aging behavior of battery cells, which greatly influences the operational capability of battery-powered devices. By combining electrochemical and analytical measurement methods, artificial intelligence (AI) and automated data processing, BATTERIEdigital® aims at the Machine Learning (ML)-based identification of critical aging processes and the prediction of the long-term degradation behavior of battery cells.
Economic, ecological and safe application of batteries
Model-based predictions that enable reliable condition determination and lifetime prediction of battery cells for different environmental and operating conditions are of great relevance for various applications. Utilities, automotive companies to waste management companies are highly interested in the development of reliable test procedures and analysis methods for battery cells. Topics such as safety, service life, warranty and environmental sustainability, which can vary greatly depending on the type and application area of the cells used, play a central role.
Parameter identification and lifetime prediction
In addition to service life prediction, the identification and prediction of underlying degradation processes that dominate the aging behavior of the cell under different operating conditions is crucial. For this purpose, a correlation must be made between the aging phenomena that occur, the physical parameters influenced by them and measurement methods that enable quantitative determination of these aging-relevant parameters.
In BATTERIEdigital® the experimental data generated with the help of these measurement methods are combined in object-oriented databases and used to train and test prediction models. For this purpose, features that show a correlation to the health status of the cell, or to certain aging processes are determined.
Ultimately, fully automated, intelligent data processing based on ML algorithms is envisioned through the creation of digital workflows. This ML approach can also be used for material development and cell manufacturing in order to derive and test suitable process parameters for the production of materials, components or cells with certain specifications.
Data preparation as a basis for machine learning models
The development of innovative strategies and efficient methods for cell chemistry-independent lifetime prediction and the identification of aging-relevant parameters is becoming increasingly important. Data-based methods use black-box models to establish a mathematical link between selected input parameters and the objective function to be modeled. In contrast to physico-chemical models, in this case no exact knowledge of the cell chemistry and the reactions occurring is required. Using classification methods, ML approaches, and intelligent optimization algorithms, BATTERIEdigital® can accurately predict target variables and reveal correlations between input parameters and target variables.
The essential basis for constructing an ML model lies in data processing (cleaning and feature engineering). The system is then trained with training data and subsequently tested with a test data set. The generation of an extensive database serves to improve the accuracy and robustness of machine learning methods.
Empirical and statistical methods are used in BATTERIEdigital® to identify relevant aging parameters, e.g. with the help of post-mortem analyses, and to validate data-based models.